Newsportal - Ruhr-Universität Bochum
AI has found its way into environmental management and agriculture
Temperatures above 35 degrees centigrade in the summer, followed by storms and torrential rain: the phenomena we’ve been experiencing in the recent years constitute a harbinger of future weather events caused by climate change. The consequences include: crop failure, river shipping being hampered due to low water, floods and storm damage in the cities.
In order to tackle climatic changes, modern solutions are required
“In order to tackle these climatic changes, modern solutions are required,” maintain Dr. Benjamin Mewes and Dr. Henning Oppel from the Institute of Engineering Hydrology and Water Resource Management at RUB. In their joint project “Okeanos”, the two engineers are deploying Artificial Intelligence. While Benjamin Mewes focuses on irrigated agriculture, Henning Oppel is figuring out how to better predict flood events.
Agriculture is the largest consumer of fresh water globally. According to the Food and Agriculture Organization of the United Nations, around 30 per cent of fresh water used is used to irrigate fields, orchards, vineyards and other agricultural land.
“Most of the time, farmers employ their expertise and experience to decide when and how to water their soil,” says Mewes. However, this results in hundreds of thousands litres of this valuable resource being wasted. This could be remedied by a computer software that provides a recommendation for action based on all the important factors for irrigation – or better still, controls the irrigation systems itself.
Today, plenty of extremely helpful environmental information is available to us.
“Today, plenty of extremely helpful environmental information is available to us; however, farmers don’t put it into context and consider it as a holistic system. We know how the water and with it the fertiliser travel through the soil, how they are absorbed by different plants, and we have pretty accurate weather forecasts and sensors that measure soil moisture,” points out Benjamin Mewes.
The good thing is that most farmers are in favour of digitalisation. “Agriculture is the most digitised industry in Europe,” explains Mewes. Automated tractors, digitally controlled feeders and drone flights over the fields: farmers have recognised the potential of modern technologies and hope to use them to reduce costs while increasing yields.
Mewes has developed an agent-based soil/water model. Agents are software units that act autonomously and make decisions in accordance with a set of rules and that can represent complex systems and chains through their interaction with each other.
In Mewes’ model, which each farmer would be able to adapt to his specific requirements, an agent represents a certain amount of water. It can move, contain fertilisers and interact with its environment, i.e. the soil. In doing so, the agent changes the environment for any subsequent agents.
A dynamic model that can be adapt to individual conditions
If, for example, the soil through which the water flows is already saturated with nutrients, the agent cannot release its fertiliser there, but only in deeper soil layers. Moreover, Mewes can implement exceptions into the model, as he explains: “If a farmer knows that there is a 40 per cent probability of hitting a mouse hole in his field, I can incorporate these into the model. This can be relevant, because water flows faster through the holes than through the surrounding soil.” Accordingly, the model is dynamic, can adapt to individual conditions, and it thus offers every farmer a tailor-made irrigation strategy.
The agents have learned how to behave in which situation through machine learning, i.e. by Mewes training them for different situations using many examples, which they then generalised. “The model supplies information about space and time, and I can use it to represent actual interaction processes. This helps us take the complex processes in the soil into account,” as Mewes outlines the advantages over conventional models.
A water management problem of a very different kind
Henning Oppel researches into a water management problem of a very different kind. He intends to pave the way for more precise flood warnings with the aid of machine learning. “This makes sense, because floods are the result of complex interactions that can easily be simulated in this way,” as he explains.
To understand this, it is necessary to first visualise how water gets into a river: when rain or snow falls, some of it flows onto roads and other sealed surfaces. The greater part of it seeps into the soil in forests and on arable land and grassy areas. A third option is the water travelling into deeper layers of the soil where it reaches groundwater.
The path of the water defines its speed. On the surface, it can reach the next body of water within a few minutes, while underground it can remain in place for several years.
Which route it takes depends not only on the land use, but also on the type of rainfall. During heavy rainfall events, a much greater proportion of the water drains off on the surface than during prolonged continuous rainfall.
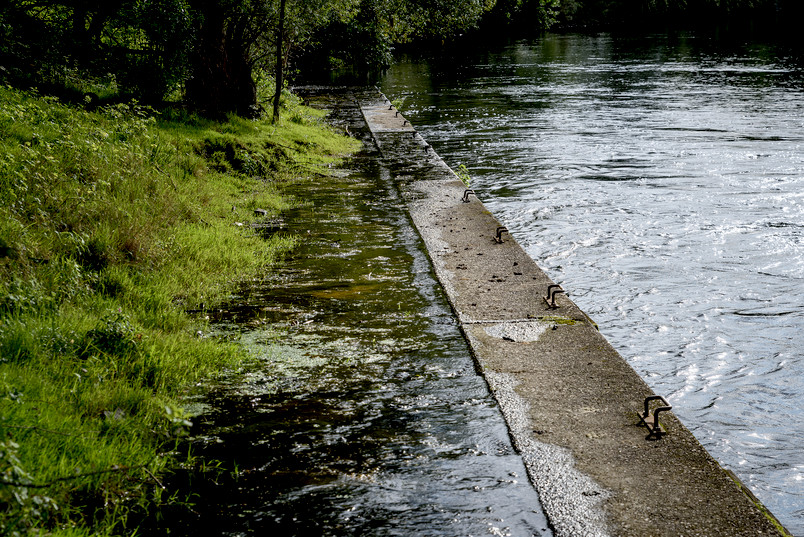
In order to predict how the water level of a river will change, it is not enough to simply consider the processes at the location for which a flood forecast is required; rather, it is necessary to take the entire area stretching behind it into consideration. It might cover the thousands of square kilometres that define a river’s catchment, including a wealth of different surfaces such as asphalt, forest soil or gravel, as water moves at different speeds on all of them. “Due to the large number of active processes, it is difficult to apply a single process equation. Still, machine learning enables us to develop new process descriptions and add to existing concepts,” says Oppel.
The data for his Artificial Intelligence are based on two values: precipitation records and water levels. He obtained these for the three rivers Upper Main, Regen and Iller, all located in Bavaria. The authorities there have been recording the values hourly since 1970.
“I establish a functional relationship between precipitation data and water level data and try to teach the AI how fast precipitation becomes river water under different conditions. It also depends on how much it rained before the event started, whether there is still snow on the ground, and a number of additional conditions,” lists Oppel.
Compared to prediction models currently in use, Henning Oppel’s model offers one considerable advantage: it takes into account the type of the expected flood event. It thus essentially consists of three models: one for very fast, one for moderately fast and one for slow water drainage. To this end, the program has to know in advance how much rain is to be expected: data that are provided by relevant weather forecasts.
Benefit for flood reporting services, insurance companies and others
“Previous models could represent only one process. If rainwater had flowed mainly near the surface towards the river in the past, then the same moderate flow velocity is also assumed for the present,” as Oppel explains the reason why flood prediction models struggle with new phenomena such as heavy rainfall events in cities caused by climate change.
A number of potential target groups might benefit from an improved solution in the form of an app: flood reporting services, insurance companies, fire brigades and technical assistance services, to name but a few.
25 September 2019
9.23 AM