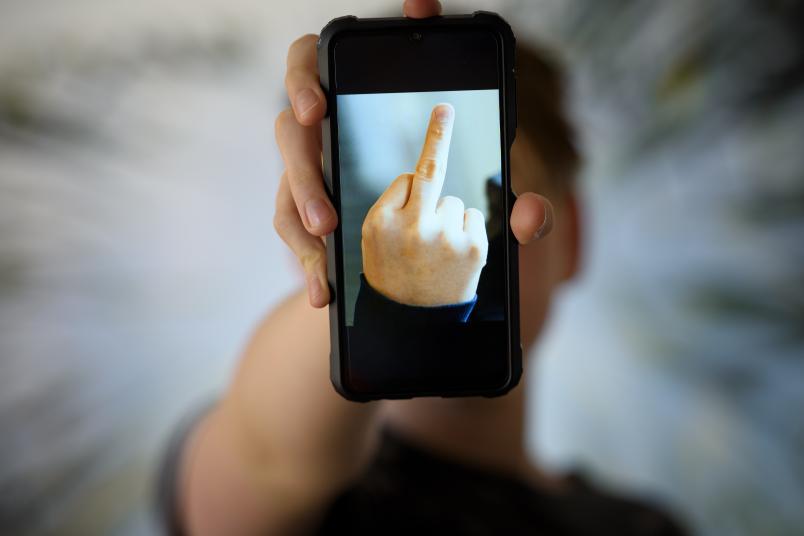
Linguistics The destructive power of language
Artificial intelligence is capable of identifying slurs. But can it detect hidden forms of verbal violence?
“Fuck off, bitch!” “I’ll hunt you down, asshole! I’ll put a knife in you!” “They should all be shot!” These are just a few examples of the form language can take on social media. People are insulted, threatened or incited to criminal behaviour. Professor Tatjana Scheffler is interested in the features of hate speech and other forms of harmful language from a linguistic perspective and in how they can be automatically detected. She works in the field of digital forensic linguistics at RUB.
“Language processing has improved immensely over the past few years,” says Scheffler. Today, users of translation programmes like Google Translator or voice assistants like Siri get much better results than they did a few years ago. The classification of texts also works pretty well now. Artificial intelligence algorithms can learn to assign statements to different categories. For example, they learn to determine whether a text passage contains a direct insult or not. The algorithms learn the categories using large training datasets that have been classified by humans. Subsequently, they can transfer the knowledge about the learned categories to new data.
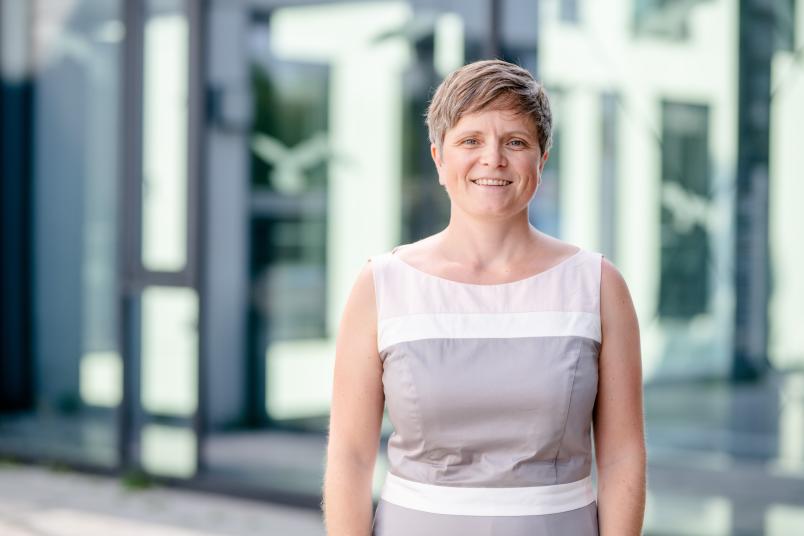
“Direct insults and swear words are easier to identify,” says Tatjana Scheffler. Often, all the algorithm has to do is to compare the data with a list of frequently used insults. According to the researcher, however, harmful language is much more than obvious hate speech directed at individuals. “There are more implicit forms that are not directed at a specific recipient,” she explains. Harm can also be done by talking about others in a certain way or by creating a certain atmosphere.”
At worst, such sentiments can turn into real violence. A famous example is the storming of the Capitol by supporters of then-US President Donald Trump on 6 January 2021. Social media is partly blamed for allowing the situation to escalate.
Telegram chat of Trump supporters analysed
This example, namely the storming of the Capitol, is precisely what Tatjana Scheffler looked into, together with two colleagues from Berlin. The group examined 26,431 messages from 521 users on the messenger service Telegram. The messages were posted between 11 December 2016 and 18 January 2021 in a public channel where people with extreme right-wing views exchanged ideas. Their discussion started with the theoretical idea of overthrowing the government and gradually developed into concrete plans to storm the Capitol.
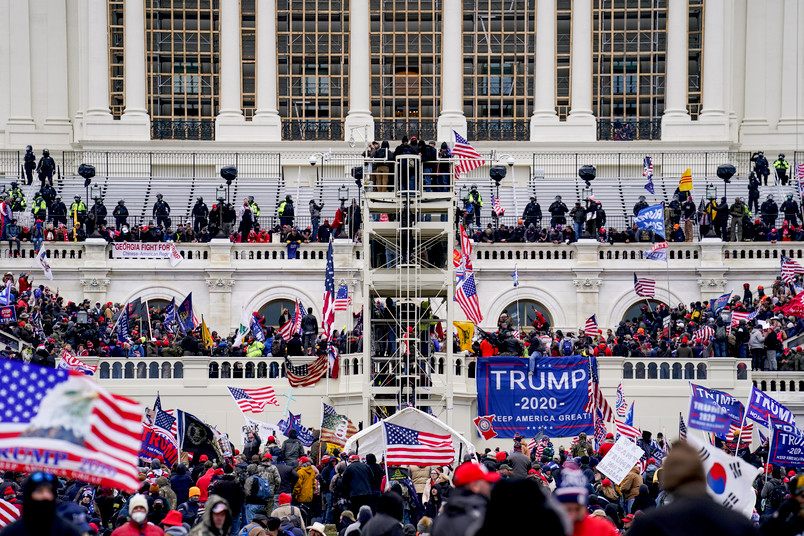
Tatjana Scheffler’s team assessed how well existing algorithms could identify harmful speech in this dataset. In order to evaluate the accuracy of the algorithms, they analysed about one fifth of the messages by hand and compared their results with those of the automated processes. They distinguished five different forms of harmful language.
Five categories of harmful language
The first category included inflammatory language such as “violence is 100000% justified now”. The second category included derogatory terms such as “scum” or “retarded”. In the third category, the team included terms that are not derogatory in themselves, but were meant to be derogatory in the context in which they occurred – such as “they are a sickness”. A fourth category was reserved for so-called othering: remarks used to distinguish one group of people from another, as in this example: “Are women banned from this chat? If not, why the fuck not?” The last category included insider phrases used by a group of like-minded people to distinguish themselves from others and strengthen their sense of community. Trump supporters, for example, use the term “patriot” in a certain way.
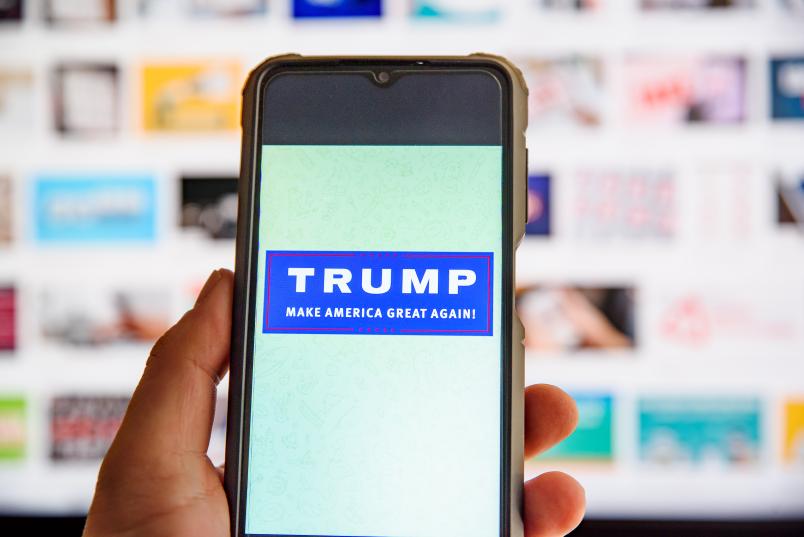
Comparing automated processes and humans
After coding the comments in this way, the researchers used automated processes to label them, such as those deployed by tech companies to detect hate speech or offensive language. 4,505 messages were included in the comparison. Of these, 3,395 were rated as non-harmful by both the researchers and the automated processes, and 275 were agreed to contain harmful language. In contrast, humans and machines assessed 835 messages in different ways: the algorithms incorrectly classified about half of them as hate speech or insults; they did not recognise the rest – unlike the researchers – as harmful language.
Certain grammatical structures can be an indication that a term is meant to be derogatory.
Tatjana Scheffler
“When we see in which cases established methods make mistakes, it helps us to create better algorithms in the future,” Tatjana Scheffler points out. Together with her team, she is also developing new automated methods to better recognise harmful speech. This requires, for one thing, better training data for the artificial intelligence. For another, the algorithms themselves also need to be optimised. This is where linguistics comes into play again: “Certain grammatical structures, for example, can be an indication that a term is meant to be derogatory,” explains Scheffler. “If I call someone ‘you twig’, that’s not the same as simply saying ‘twig’.”
Improving algorithms
Tatjana Scheffler is looking for such linguistic features to feed the algorithms of the next generation with deeper background knowledge. Contextual information, too, could help the machines find harmful language. What kind of person left the comment? Have they made derogatory comments about others before? Who is being addressed – a politician or a journalist? These groups are most likely to be subject to verbal attacks. Such information could also increase the accuracy of an artificial intelligence.
It won’t work without the expertise of humans.
Tatjana Scheffler
Tatjana Scheffler is convinced that automatic methods are necessary to tackle the problem of harmful language. The sheer number of comments is too large for humans to sift through and evaluate them all without support. “But it won’t work without the expertise of humans,” the researcher emphasises. After all, there will always be cases where the machines are wrong or not sure.
Original publication