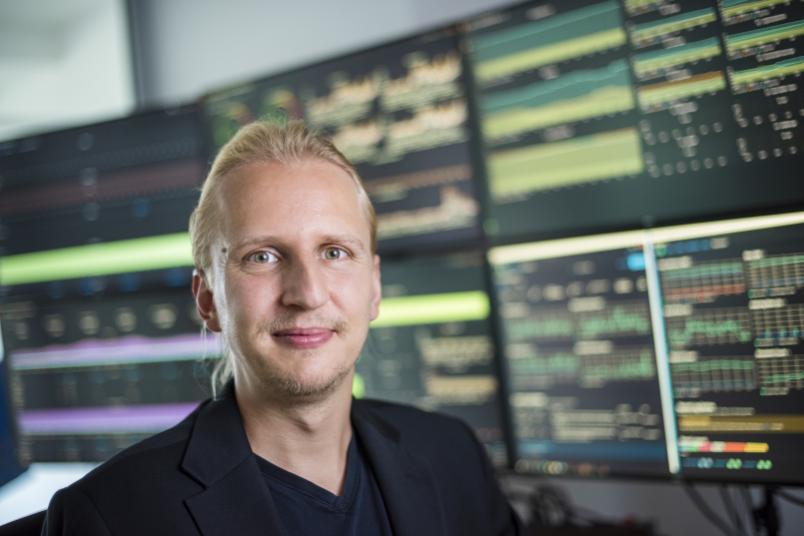
Neuroinformatics Making models bounce
Machines can be taught to reliably detect cancer. What we need now are methods to ensure data protection. Researchers in Bochum already have an idea of how this can be achieved.
Computer, can you identify a carcinoma in the thorax scan? Show me all patients with similar diagnostic findings! What sounds futuristic is already common practice in many smart hospitals. Doctors click through databases at lightning speed, make diagnoses or compare scans – thanks to the early versions of artificial intelligence (AI). But can these systems automatically detect cancer, too? “The most advanced neural networks are really good. They can detect cancer more reliably than doctors,” says Dr. Michael Kamp from the RUB Institute for Neuroinformatics.
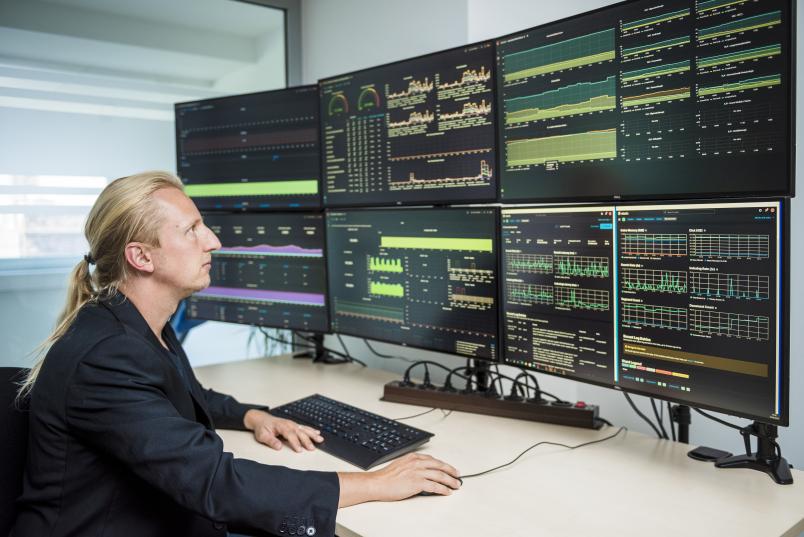
In cooperation with the Institute for Artificial Intelligence in Medicine (IKIM) at Essen University Hospital, Kamp is exploring how artificial intelligence systems can be trained for the sensitive field of medicine using machine learning methods, most importantly deep learning. The specific learning technique that Kamp’s research group Trustworthy Machine Learning has specialised in and intends to optimise is called federated learning. It is ideally suited to meet the high demands that medicine places on AI.
Machine learning
“Let’s say one hospital has images of 50 patients with a certain type of cancer and another has 40 scans of other patients. Due to data protection, they are of course strictly prohibited from sharing such sensitive patient data. But in order to train neural networks, we need all this data in one place,” as Kamp describes the challenge his team faces. How can confidential data that is not allowed to leave the hospital still be made usable? This is where the technique of federated learning comes in.
Federated learning
“For federated learning, we first train a model in each hospital with the locally available data in a decentralised manner, until the neural network at that hospital reaches a level where it can recognise a certain type of cancer,” explains Kamp. What does the training look like in practice? How do you teach machines to recognise cancer? “The computer learns to derive a pattern or rule from images, such as CT scans. To this end, it resolves the individual image pixels into data and then calculates a result using a mathematical formula. It then compares this with the description of the scan. It repeats this process many thousands, often millions of times, until it can make a relatively reliable prediction as to whether a scan shows a carcinoma or not,” elaborates Kamp.
Sending models on tour
The abstracted model created after the training sessions at one hospital can then be sent to the next hospital. “The basic architecture of the models is the same everywhere, because the underlying problem – the detection of a certain type of cancer – is the same,” points out Kamp. The patients’ data stays behind at the hospital; only the samples go travelling. In the next clinic or doctor’s practice, the learning process is repeated with new scans. The more data are fed into the network, the better it can later distinguish and evaluate the datasets. The advantage of the learning technique is: the hospitals feed the model without sharing their data. If many models are sent travelling at the same time, they are brought together after a while and combined into an exceptionally high-quality model.
Benefits for smaller practices
Michael Kamp’s team has found that the technique of federated learning can also work if a model is initially fed from only a few data, such as eight images per hospital. In order to ultimately obtain a reliable final model, the local model has to rotate several times from hospital to hospital before it is combined with others to form a global model. “Only when a single model has been bounced ten times will we end up with an aggregated model that can be relied on,” as Kamp summarises the current state of research on this extended form of federated learning.
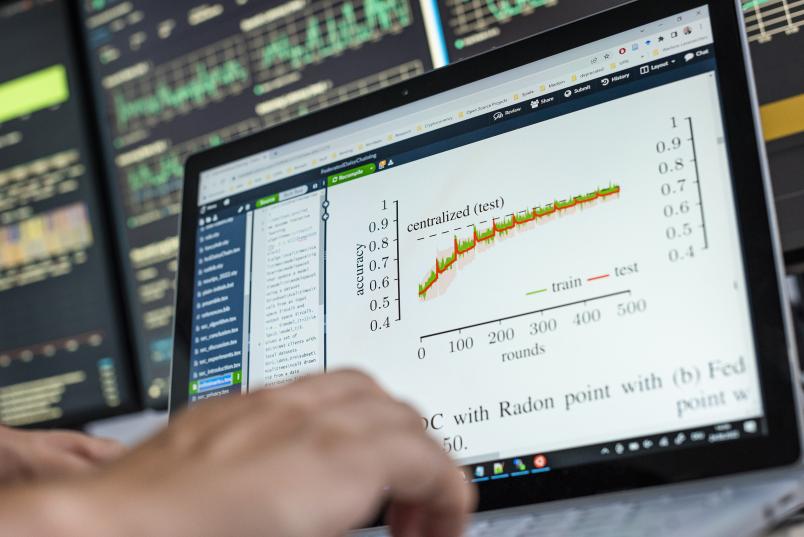
The researchers hope that, in the future, small practices or hospitals in remote areas will thus be able to participate in federated learning, ultimately benefitting from the wealth of data from larger clinics without the data leaving their original locations. Augmented federated learning appears to have many advantages for the application of AI in the field of medicine. But as the networks and models are being built, they also raise questions about quality, privacy and practicability, for example. Kamp and his team are addressing these questions in various subprojects.
Understanding the basics
First of all, the RUB computer scientists hope to find out how to guarantee good model quality for neural networks. “We ask ourselves: How can we guarantee that our neural networks will be qualitatively good, that the statements will ultimately be correct? Can we state probabilities or predict uncertainties?” says Kamp. To this end, the researchers are delving deep into the basics of learning theory. They are exploring the required properties of the data with which the models are fed and how these properties can be measured. “The challenge and the key question of learning theory is to minimise the generalisation error, i.e. the difference between the error the model makes with the data we have and the error the model makes with new, unknown data,” stresses Kamp.
Ensuring confidentiality
In addition to the basic learning technique, the researchers also intend to optimise the practical applicability of the neural networks. For example, Kamp and his team aim to ensure that confidential patient data is effectively protected when models are transferred from hospital to hospital. “It must be impossible to discover or reconstruct correlations between the models and data. They mustn’t allow any conclusions to be drawn,” stresses Kamp.
Passing the field trial
Another project team is working on rendering the finished networks or assistance systems usable and operable for the clinic staff. “At the end of the day, it must be possible for doctors to interpret them,” says Kamp. “Neural networks are complex, and it’s a challenge to find the sweet spot between too much and too little information.” In the best case, the systems make recommendations and provide high-quality explanations and justifications straight away.
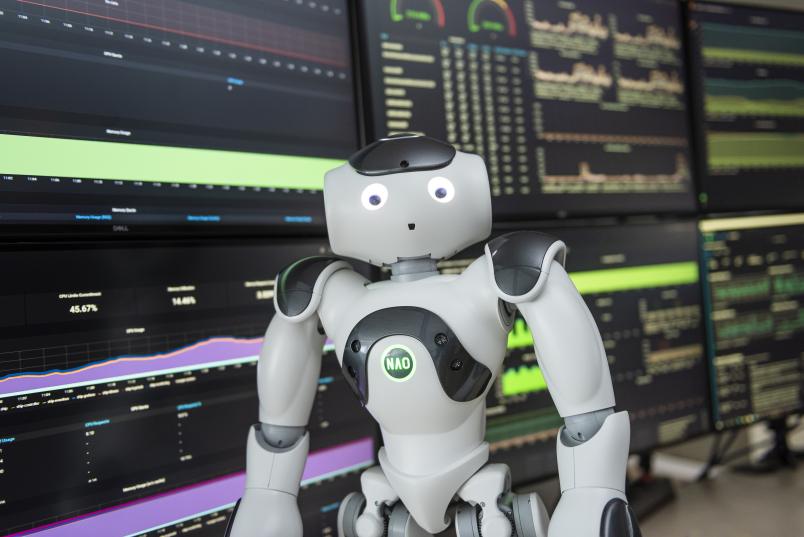
In just a few years, artificial systems will be able to assist hospital staff to an even greater extent. Perhaps assistance systems will also be able to combat problems such as the shortage of staff in rural areas. And yet, Kamp is certain that the profession of physician will remain irreplaceable: “There will always be a need for people to confirm the diagnosis made by an assistance programme. We are not rebuilding brains here, but solving a mathematical optimisation problem.”