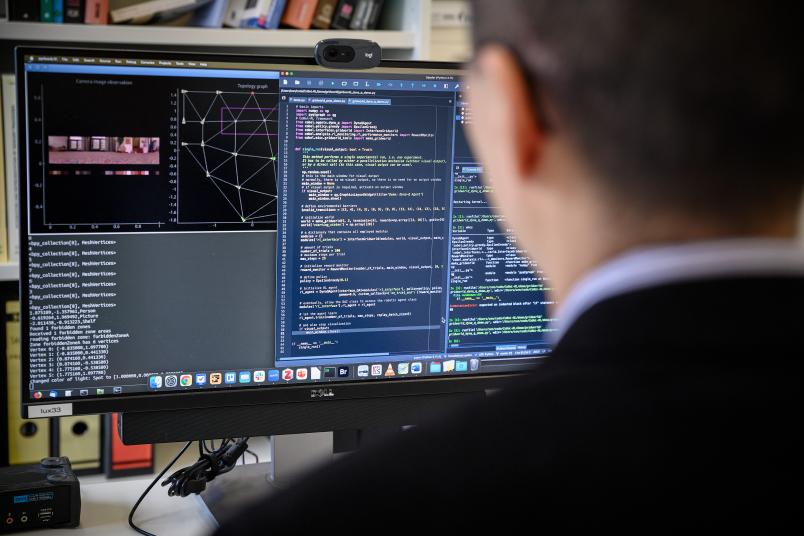
Interview Understanding the Brain Thanks to Artificial Intelligence
Computer models of neural networks developed by humans can be arbitrarily far removed from reality. Nevertheless, they are a great help to researchers in planning and evaluating learning experiments.
Why is it so difficult to let go of learned behaviors? How do computer models based on artificial intelligence (AI) help to understand this better? Computational neuroscientist Professor Sen Cheng from Ruhr University Bochum and psychology Professor Metin Üngör from Philipps University Marburg explain in a joint interview. They collaborate in the Collaborative Research Centre Extinction Learning.
Professor Üngör, what is extinction learning?
Metin Üngör: It comprises everything that ensures that learned behavior diminishes and is no longer displayed: It is extinguished.
Why is it so difficult to change existing behaviors?
Üngör: This is actually a good evolutionary adaption mechanism. I probably save energy if I don’t throw something that took effort to learn overboard as soon as the situation changes. That way it remains ready to be accessed if it changes again.
Sen Cheng: Our brain also doesn’t work like a hard drive. We cannot find and delete files on it. The brain stores information by neural networks changing their connections. The information is then distributed across these networks. This is why it probably isn’t possible to completely extinguish something that has been learned.
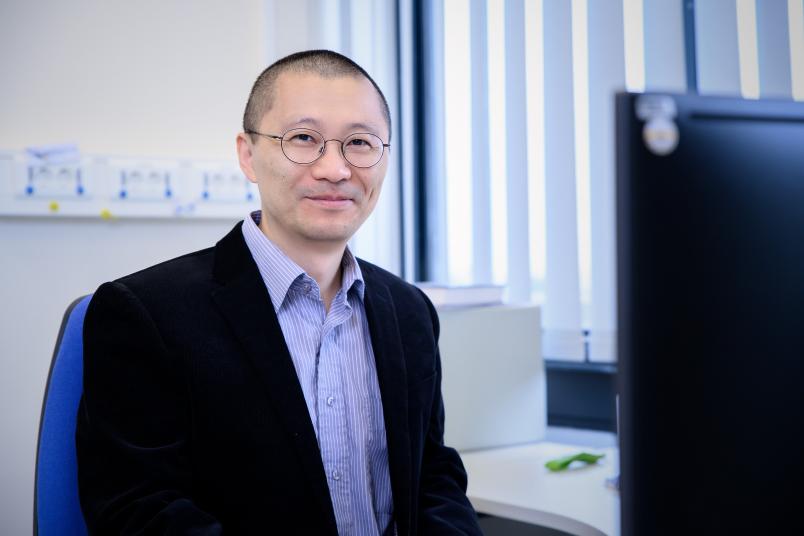
What tasks has AI taken over in your research?
Cheng: It is able to generate meaningful information from huge quantities of data. If a neuron is activated in the brain, it sends out an electrical signal, an action potential. If I detect these signals from a thousand individual neurons, I get a thousand lines.
On each of them I see the individual action potentials of a neuron as an upward swing. I then have to find meaning in it. AI can visualize how the entire population of a thousand neurons behaved in the experiment.
You can also view AI as a model system that is capable of solving complex problems. I conduct a lot of research in the field of spatial navigation. If my artificial system learns to navigate in an environment, I can see precisely what is happening in its replicated neural networks.
Isn’t it better to see this with experimental methods with which brain activity can be measured directly?
Cheng: We compare the AI data with the neural activity that we record from the brains of rats and mice when they learn spatial navigation. However, the AI offers much better access to what is happening. Because I know what algorithm it is using and can measure the activity in all units of its network simultaneously.
If I better understand the artificial system, I hope to also better comprehend the biological one.
This allows ideas to be tested and mechanisms to be researched very quickly. If I better understand the artificial system, I hope to also better comprehend the biological one. We naturally also use AI to analyze this synthetic data. We develop analysis methods and strategies that can then be applied to experimental data.
What insights do you gain when you test an assumption using AI?
Cheng: Our AI models are invented by humans and can be arbitrarily far removed from reality. Either I have to discard my assumption or I can say that there is probably something to it. We now have a rough idea of which area of the brain does what. But how exactly the activity of the different neurons causes a certain behavior to happen is still not well understood.
In my AI model, I have different types of neurons and can test whether the model behaves as expected when I activate a certain population of them. This enables me to try things out and get an idea of what I should look at more closely.
In which research topic are you currently using AI?
Cheng: In learning. It’s standard to look at behavior before and after learning. However, this gives you a view that perhaps has very little to do with reality. Learning curves are a good example of this.
If an individual learns quickly, their learning curve climbs quickly; if they learn slowly, it climbs slowly. If you average the learning curves of many people, the result no longer says anything about how an individual learns. Then it looks as though you’re always learning a little bit at a time, until you eventually don’t get any better.
But that’s not how learning really works?
Cheng: Apparently, learning does not take place gradually but instead in spurts. At some point, you go from zero to a hundred. This has nothing to do with intelligence. We see this in our models, which have very simple learning rules.
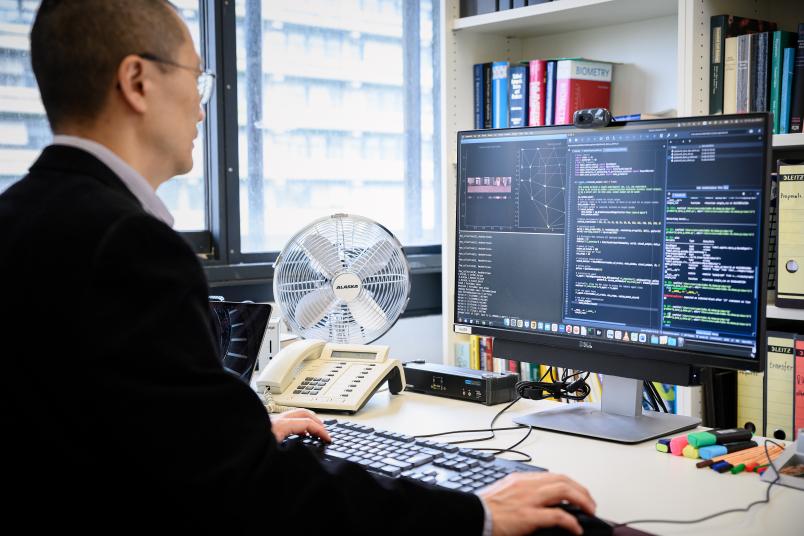
If you connect enough units together in a network, the network suddenly behaves very unexpectedly. It learns things in just one attempt. Although the individual parts only learn associations and change very little locally, this can add up in a non-linear way throughout the system and lead to sudden changes in behavior.
We also observe these spurts, after which behavior changes abruptly, and extreme differences between individuals in humans, rats and pigeons. But when you look in textbooks, you always see averaged gradual learning curves.
Do other scientists also use your AI models?
Cheng: There are some who would perhaps like to use them, but we first have to show to what extent they work and deliver insights that we would not otherwise have.
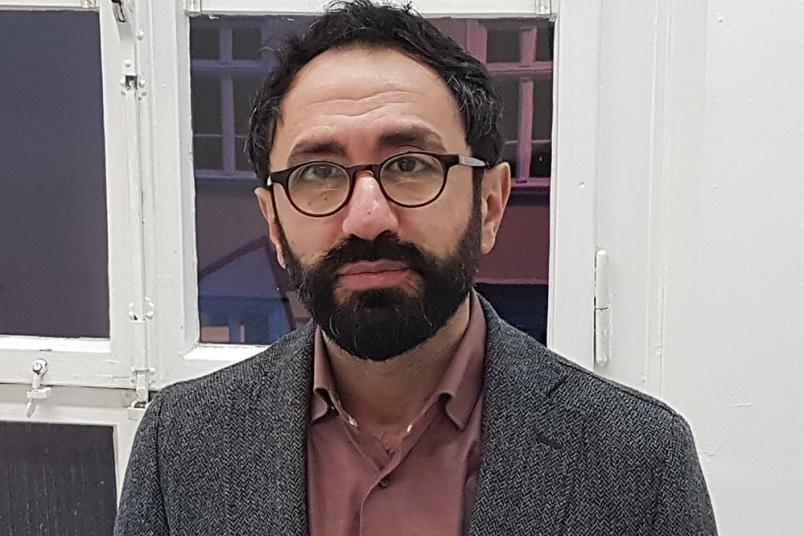
Üngör: In our Collaborative Research Center, many researchers already use experimental designs that we have developed with support from AI. These can then be implemented in human experiments with relatively simple experimental arrangements, such as predictive learning tasks. Predictive learning tasks are special categorization tasks that have been used in experiments for decades.
For example, the participants in our experiment take on the role of a doctor whose patient is suffering from abdominal pain. The doctor now needs to find out which foods the patient has an allergic reaction to and which are harmless to them. In the experiment, the doctor gives the patient various things to eat and can directly observe how they react to them. Tasks such as this can be adapted to many research questions and thus very quickly create specific learning experiences for the participants, which can then be changed over the course of the experiment.
What role does AI play in this?
Üngör: In an experiment, we often want to test two competing theories against each other that explain something via different mechanisms. To do this, we create situations in which both theories lead to different predictions on how a test subject will behave. Simulations can help to reduce the number of experiments necessary.
These predictive learning experiments in human research are increasingly replacing animal testing.
Do you still need experimental animals for these experiments?
Üngör: These predictive learning experiments in human research are increasingly replacing animal testing. There are more and more research groups that used to work purely with animal testing that have since either switched completely to human research or use human experiments alongside animal testing.
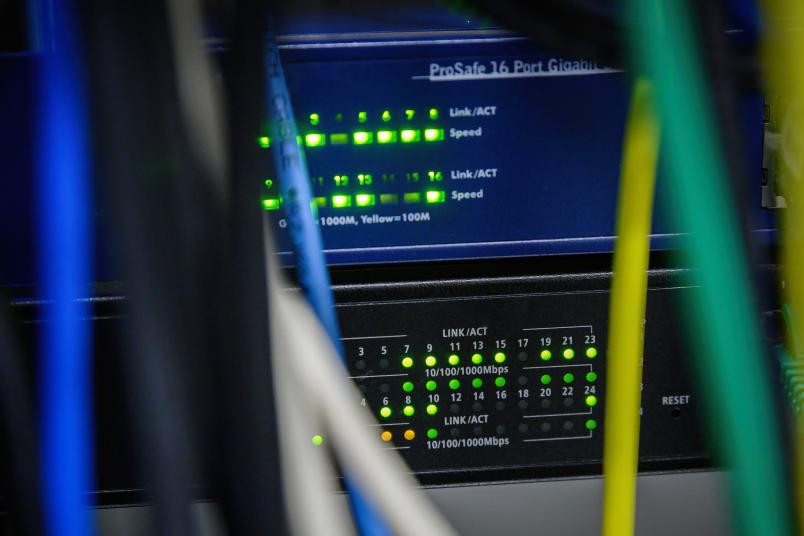
Cheng: This may reduce the need for experiments on animals that would otherwise be carried out to find out which areas of the brain are important and which are not. I can also try out in advance how many neural derivations are needed to get a meaningful result in the experiment.
Will it eventually be possible to understand the function of the entire human brain?
Cheng: I believe that we will one day be able to do this, but presumably in the distant future. There are many things that my brain does not comprehend, but I can program these things and run them as a simulation. I then draw my conclusions from this. Viewed in this way, AI can perhaps even help me to understand my brain by preparing the data in a way that I can understand.