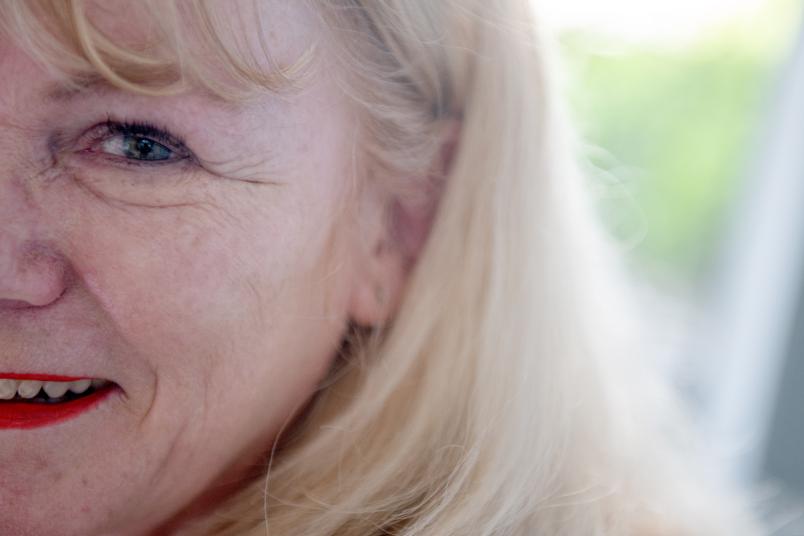
Artificial intelligence The benefits of slowness
An algorithm developed by neuroinformatics engineers in Bochum estimates age and ethnic origin as exactly as humans do. The researchers are not yet sure which features it interprets.
Wrinkles, furrows, spots: a person’s aging process is accompanied by tell-tale signs on their face. Researchers from the Institute for Neural Computation at Ruhr-Universität Bochum (RUB) have developed an algorithm that interprets these features very reliably. It makes it possible to estimate the age and ethnicity of people so accurately that it catapulted RUB researchers to the top of the league table worldwide for a while. The RUB team published its report in the journal “Machine Learning” from May 2020.
The system has learned to estimate
“We’re not quite sure what features our algorithm is looking for,” says Professor Laurenz Wiskott from the Institute for Neural Computation. This is because the system has learned to assess faces. The successful algorithm developed by the Bochum-based researchers is a hierarchical neural network with eleven levels. As input data, the researchers fed it with several thousand photos of faces of different ages. The age was known in each case. “Traditionally, the images are the input data and the correct age is the target fed into the system, which then tries to optimise the intermediate steps to assess the required age,” explains lead author Alberto Escalante.
However, the researchers from Bochum chose a different approach. They input the many photos of faces sorted by age. The system then ignores the features that vary from one picture to the next and takes solely those features into consideration that change slowly. “Think of it as a film compiled of thousands of photos of faces,” explains Laurenz Wiskott. “The system fades out all features that keep changing from one face to the next, such as eye colour, the size of the mouth, the length of the nose. Rather, it focuses on features that slowly change across all faces.” For example, the number of wrinkles slowly but steadily increases in all faces. When estimating the age of the people pictured in the photos, the algorithm is only just under three and a half years off on average. This means that it outperforms even humans, who are real experts in face recognition and interpretation.
The system also recognises ethnic origins
The slowness principle also enabled it to reliably identify ethnic origin. The images were presented to the system sorted not only by age, but also by ethnicity. Accordingly, the features characteristic of an ethnic group didn’t change quickly from image to image; rather, they changed slowly, albeit by leaps and bounds. The algorithm estimated the correct ethnic origin of the people in the photos with a probability of over 99 percent, even though the average brightness of the images was standardised and, consequently, skin colour wasn’t a significant marker for recognition.