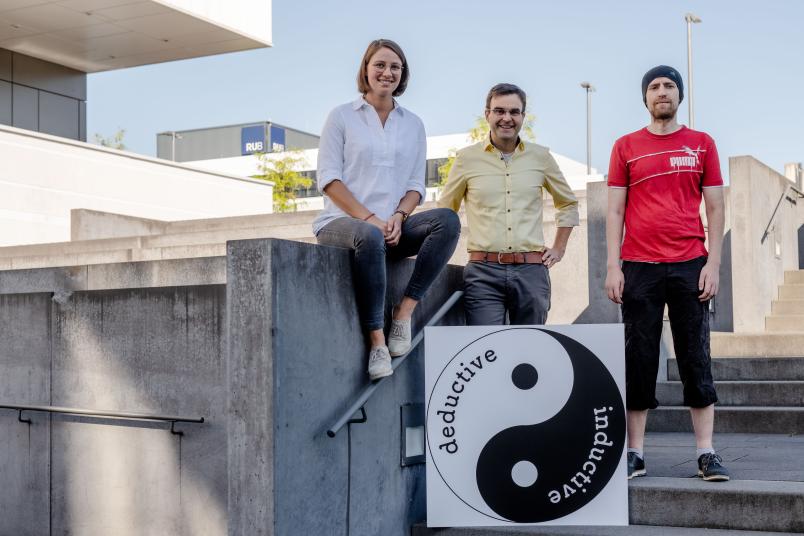
Bioinformatics
How artificial intelligence can explain its decisions
Today, when an algorithm identifies a tumour in a tissue sample, it doesn’t reveal how it arrived at this result. And that’s not very trustworthy. Researchers in Bochum are therefore pursuing a new approach.
Artificial intelligence (AI) can be trained to recognise whether a tissue image contains a tumour. However, exactly how it makes its decision has remained a mystery until now. A team from the Research Center for Protein Diagnostics (PRODI) at Ruhr-Universität Bochum is developing a new approach that will render an AI’s decision transparent and thus trustworthy. The researchers led by Professor Axel Mosig describe the approach in the journal “Medical Image Analysis”, published online on 24 August 2022.
For the study, bioinformatics scientist Axel Mosig cooperated with Professor Andrea Tannapfel, head of the Institute of Pathology, oncologist Professor Anke Reinacher-Schick from the Ruhr-Universität’s St. Josef Hospital, and biophysicist and PRODI founding director Professor Klaus Gerwert. The group developed a neural network, i.e. an AI, that can classify whether a tissue sample contains tumour or not. To this end, they fed the AI a large number of microscopic tissue images, some of which contained tumours, while others were tumour-free.
“Neural networks are initially a black box: it’s unclear which identifying features a network learns from the training data,” explains Axel Mosig. Unlike human experts, they lack the ability to explain their decisions. “However, for medical applications in particular, it’s important that the AI is capable of explanation and thus trustworthy,” adds bioinformatics scientist David Schuhmacher, who collaborated on the study.
AI is based on falsifiable hypotheses
The Bochum team’s explainable AI is therefore based on the only kind of meaningful statements known to science: on falsifiable hypotheses. If a hypothesis is false, this fact must be demonstrable through an experiment. Artificial intelligence usually follows the principle of inductive reasoning: using concrete observations, i.e. the training data, the AI creates a general model on the basis of which it evaluates all further observations.
The underlying problem had been described by philosopher David Hume 250 years ago and can be easily illustrated: No matter how many white swans we observe, we could never conclude from this data that all swans are white and that no black swans exist whatsoever. Science therefore makes use of so-called deductive logic. In this approach, a general hypothesis is the starting point. For example, the hypothesis that all swans are white is falsified when a black swan is spotted.
Activation map shows where the tumour is detected
“At first glance, inductive AI and the deductive scientific method seem almost incompatible,” says Stephanie Schörner, a physicist who likewise contributed to the study. But the researchers found a way. Their novel neural network not only provides a classification of whether a tissue sample contains a tumour or is tumour-free, it also generates an activation map of the microscopic tissue image.
The activation map is based on a falsifiable hypothesis, namely that the activation derived from the neural network corresponds exactly to the tumour regions in the sample. Site-specific molecular methods can be used to test this hypothesis.
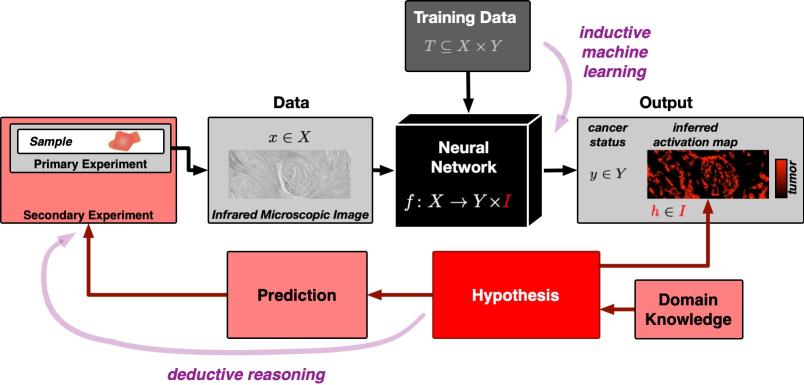
“Thanks to the interdisciplinary structures at PRODI, we have the best prerequisites for incorporating the hypothesis-based approach into the development of trustworthy biomarker AI in the future, for example to be able to distinguish between certain therapy-relevant tumour subtypes,” concludes Axel Mosig.