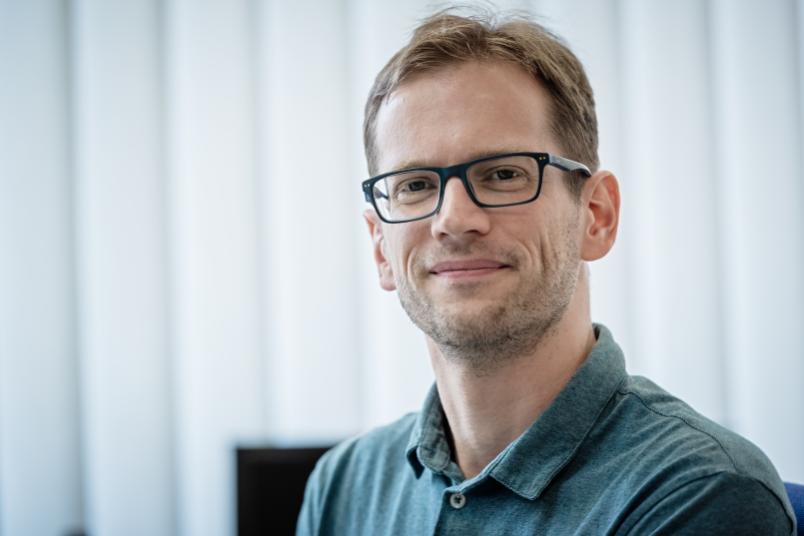
Point of view When machines research
Self-learning computers won’t put researchers out of work. That’s because what they lack is a healthy dose of human scepticism.
Since the early 17th century, research has been an interplay between prediction and contradiction. Theoretical research attempts to describe a context that had previously not been understood using analogies or, where possible, mathematical models. Experimental research strives to transfer and confirm those descriptions for more general cases. If the predictions do not agree with the experimental results, the theoretical description must be adapted or replaced, and the whole process starts over. Although the tools used to develop and test theories have evolved considerably since those days, this principle had not changed for a long time.
Machine learning is an established field of computer science, which has recently been attracting more and more attention following a number of innovative technical applications. The objective is to automatically derive mathematical descriptions by analysing data: how to describe the pixels on a camera chip when capturing a traffic sign, vehicle, cyclist or pedestrian? How to describe the sound waves that are recorded when someone says “Alexa” or “OK, Google”? How to describe the probability of winning a game of Go with a given arrangement of stones?
Because of new programs that penetrate our everyday lives, we often consider machine learning applications intelligent filters, detectors for changeable and sometimes highly complicated patterns, with which we can now automatically and efficiently search other data sources such as images and sound instead of text. In fact, even small research projects are now able to collect and process data that used to require humans to perform many routine operations, and which can thus explore more comprehensive and complex interrelationships.
Machine learning systems can represent both sides of the research interplay
What this development truly means exceeds public perception: not only can automatically developed high-dimensional mathematical relationships be much more complex than ever before. The methods also make it possible to identify less confident, i.e. presumably poorly predicted, cases and to automatically propose new data points for research in the form of new experiments. In other words, machine learning systems can represent both sides of the research interplay to some extent: prediction and contradiction.
However, scientists should by no means start looking for a new career. The relationships uncovered by machine learning systems can often be complex, barely comprehensible, just plain wrong or not generalisable, and they currently still elude our theoretical explanation. The methods describe without understanding. Accordingly, a healthy dose of human scepticism will remain the foundation of any science.