Neuroinformatics Driving school for computers
In order to generate realistic images of road signs, researchers pit two algorithms against each other.
To ensure that cars will one day drive autonomously and safely through the streets, they must be able to recognise road signs. Even at night, in the rain, in the snow, or if the signs are covered in moss, dirty or partially overgrown. In order to learn how to do that, they require a plethora of examples of all road signs from different seasons, times of day and weather conditions. “Taking pictures of all those signs somewhere would be immensely time-consuming,” explains Professor Sebastian Houben from the RUB Neural Computation Institute. “Especially since some of the signs are quite rare.”
Together with Dominic Spata and Daniela Horn, he therefore developed a method to generate traffic signs automatically that computers can use to practise vision.
Machine-based processes are better at recognising the signs than humans
In its infancy, the project used pictures of real road signs: back in 2011, the team took videos of 43 road signs standardised in Germany – researchers refer to them as classes. Based on the videos, they generated approximately 50,000 individual images of the signs from different perspectives. Machine-based processes are on the whole better at recognising the signs in those images than humans: the latter identified 98.8 per cent correctly, whereas an image recognition software is correct in up to 99.7 per cent of the cases.
But this is no longer the main issue. “We want to reach a point where an algorithm learns to generate images of road signs that other programs can use to practise their recognition capabilities,” outlines Sebastian Houben.
The two algorithms are sparring partners, of sorts.
Sebastian Houben
The research team uses two algorithms for this purpose: one is fed simple iconographic pictograms of official road signs and is given the task to transfer them into images that look like photos; plus, the algorithm must be able to also transfer the obtained sign back into those pictograms at a later point. “This is how we prevent the algorithm from distorting the image of the sign to such an extent that it no longer resembles the road sign in any way,” explains Daniela Horn.
The second algorithm has to decide if the generated image is a real photo or not. The goal is to ensure that the second algorithm can no longer tell what it is. “Moreover, the second algorithm indicates to the first one in what way the selection process could be made even more difficult,” says Sebastian Houben. “Accordingly, these two are sparring partners, of sorts.”
After two or three days, we check what the pictures of road signs look like.
Daniela Horn
At first, the training process doesn’t work particularly well. It counts as a success if the picture of a priority road sign has the right colour and is more or less square. But it’s improving apace. “After two or three days, we check what the pictures of road signs look like,” explains Daniela Horn. “If the pictures don’t look good to our human eye, we modify the algorithm.”
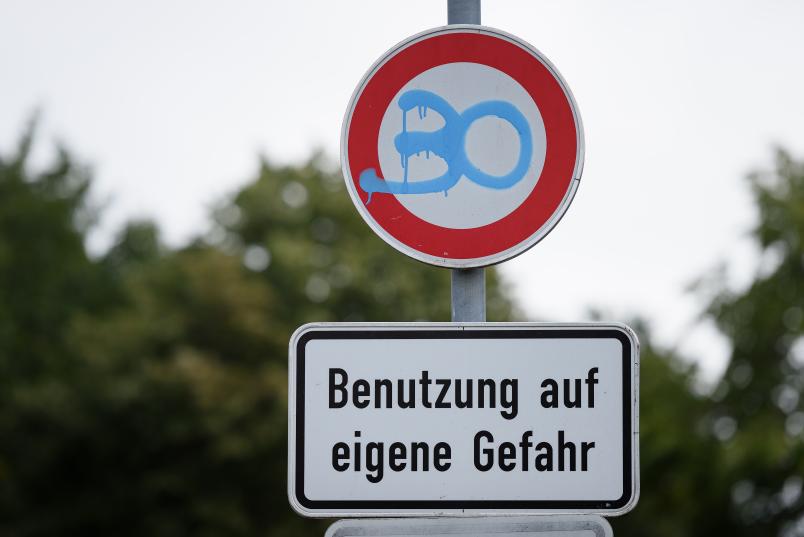
It’s not quite clear when the process will be completed, because a definite measure of image quality doesn’t exist. Human participants are fooled by only ten per cent of images on average that were created using high-quality image-generating processes. In most cases, humans recognise which images are real photos and which are not. “The reasons might be quite simple,” says Daniela Horn. “There was one case, for example, where the algorithm would always omit the pole on which a sign is mounted.”
It is not about deceiving humans
For humans, this is an obvious criterion, for a computer system not important at all. “This is not about deceiving humans,” points out the neuroinformatician. In terms of image recognition software, the two algorithms achieved better results than humans, too: following training with a comparable number of artificial images, a visual computer system performed merely ten percentage points more poorly than after training with real images.
The research team is moreover using tricks to optimise the image-generating algorithm. “It had, for example the tendency to create forest backgrounds – presumably because the image recognition algorithm is easily fooled by them,” elaborates the researcher. The team tackled this problem by changing the background colour of the original pictograms. “We can influence the process only through the initial input and by modifying the algorithm,” says Sebastian Houben. The subsequent decisions made by the algorithms are outside the researchers’ control – a feature of artificial intelligence.